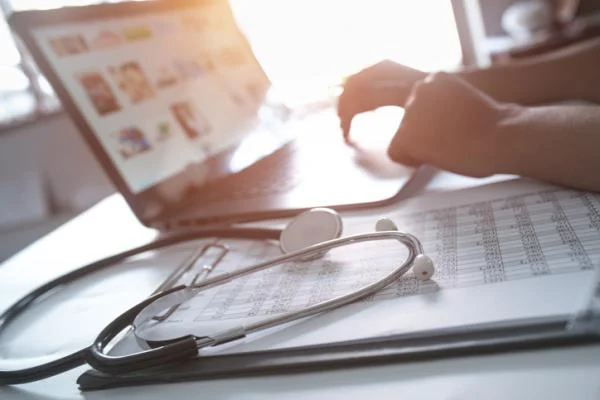
Getty Images
October 13, 2023 — Locally run large language models (LLMs) may be a feasible option for extracting data from text-based radiology reports while preserving patient privacy, according to a new study from the National Institutes of Health Clinical Center (NIH CC) published in Radiology, a journal of the Radiological Society of North America (RSNA). LLMs are deep-learning models trained to understand and generate text in a human-like way.
Recently released LLM models such as ChatGPT and GPT-4 have garnered attention. However, they are not compatible with healthcare data due to privacy constraints.
“ChatGPT and GPT-4 are proprietary models that require the user to send data to OpenAI sources for processing, which would require de-identifying patient data,” said senior author Ronald M. Summers, M.D., Ph.D., senior investigator in the Radiology and Imaging Sciences Department at the NIH. “Removing all patient health information is labor-intensive and infeasible for large sets of reports.”
In this study, led by Pritam Mukherjee, Ph.D., staff scientist at the NIH CC, researchers tested the feasibility of using a locally run LLM, Vicuna-13B, to label key findings from chest X-ray reports from the NIH and the Medical Information Mart for Intensive Care (MIMIC) Database, a publicly available dataset of de-identified electronic health records.
“Preliminary evaluation has shown that Vicuna, a free publicly available LLM, approaches the performance of ChatGPT in tasks such as multi-lingual question answering,” Dr. Summers said.
The study dataset included 3,269 chest X-ray reports obtained from MIMIC and 25,596 reports from the NIH.
Using two prompts for two tasks, the researchers asked the LLM to identify and label the presence or absence of 13 specific findings on the chest X-ray reports. Researchers compared the LLM’s performance with two widely used non-LLM labeling tools.
A statistical analysis of the LLM output showed moderate to substantial agreement with the non-LLM computer programs.
“Our study demonstrated that the LLM’s performance was comparable to the current reference standard,” Dr. Summers said. “With the right prompt and the right task, we were able to achieve agreement with currently used labeling tools.”
Dr. Summers said LLMs that can be run locally will be useful in creating large data sets for AI research without compromising patient privacy.
“LLMs have turned the whole paradigm of natural language processing on its head,” he said. “They have the potential to do things that we've had difficulty doing with traditional pre-large language models.”
Dr. Summers said LLM tools could be used to extract important information from other text-based radiology reports and medical records, and as a tool for identifying disease biomarkers.
“My lab has been focusing on extracting features from diagnostic images,” he said. “With tools like Vicuna, we can extract features from the text and combine them with features from images for input into sophisticated AI models that may be able to answer clinical questions.
“LLMs that are free, privacy-preserving, and available for local use are game changers,” he said. “They're really allowing us to do things that we weren't able to do before.”
For more information: www.rsna.org
Related Artificial Intelligence Content:
AiMed Global Summit 2023 to Focus on “Changing Healthcare One Connection at a Time” in San Diego
AiMed Global Summit’s Lineup Announced
Making AI an Integral Part of the Solution
AiMed 2023: Changing Healthcare One Connection at a Time
Find more AiMed23 conference coverage here
The Pros and Cons of Using ChatGPT in Clinical Radiology: An Open Discussion
ChatGPT Passes Radiology Board Exam
JNM Explores Potential Applications for ChatGPT in Nuclear Medicine and Molecular Imaging
New Research Suggests AI Image Generation Using DALL-E 2 has Promising Future in Radiology