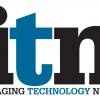
Greg Freiherr has reported on developments in radiology since 1983. He runs the consulting service, The Freiherr Group.
Will Artificial Intelligence Find a Home in PACS?
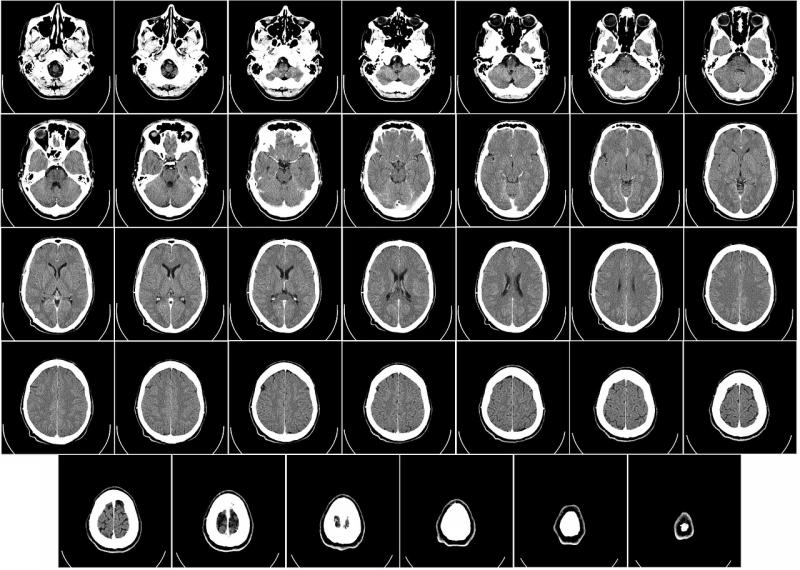
Images courtesy of Pixabay.
Time is brain. The longer a brain injury goes untreated, the higher the chance of permanent damage or death. And no brain injury is more insidious than the ones that cause hemorrhage. If unchecked, intracranial hemorrhage (ICH) and the edema that goes with it literally squeeze the brain to death.
Although ICH accounts for no more than 13 percent of all strokes, it claims 20,000 lives annually in the United States. And it comes not just from stroke but also blunt force trauma, the kind that often accompanies car accidents and assaults.
Recognizing this condition is a high priority in emergency medicine and, consequently, at vRAD, which interprets medical images for thousands of ERs across the U.S. On average, the results of emergency computed tomography (CT) of patients with intracranial bleeds are distributed, read and interpreted by vRAD neuroradiologists in 10 to 15 minutes.
A smart algorithm, stoked with artificial intelligence, promises to cut that time in half.
vRAD, also known as Virtual Radiologic (an affiliate of MEDNAX), is grooming the currently experimental algorithm as a traffic cop for its picture archiving and communication system (PACS) workflow. The algorithm, which has been taught to recognize the signs of intracranial bleeding in CT brain scans, is being trained to direct suspicious images to vRAD’s neuroradiologists.
Shannon Werb, vRAD chief information officer, explains that the algorithm, working with vRAD’s PACS, is being developed to channel the images to neuroradiologists licensed to practice in the state where the patient was scanned. The first of these neuroradiologists to become available would immediately open the study. This could cut the time elapsed from exam to interpretation from the current 10 to 15 minutes to 5 or 10 minutes, Werb said.
But before the algorithm can enter day-to-day operation, it must pass review at the U.S. Food and Drug Administration (FDA). The company is now preparing an FDA application, proposing to use the algorithm to smarten up its already FDA-cleared PACS. The algorithm’s patent filing will provide the basis for the application, he said.
Unlike other AI applications being developed as diagnostic assistants, the vRAD algorithm is not about changing the practice of medicine. It is about making medicine more efficient, according to Werb.
“This is not about replacing doctors,” he said. “We want to help physicians do a better job and do it more rapidly and more accurately.”
The algorithm will leave the practice of medicine to vRAD’s legion of radiologists, who provide preliminary interpretations during the day, and specialty and after-hours final interpretations.
Contracting for these services are about 40 percent of the healthcare facilities in the United States, according to Werb, as well as facilities in ten more countries.
The company orchestrates the interpretation of client images by assigning patient cases to the worklists of its radiologists. Assignments are made on the basis of radiologists’ special training or availability.
“We do this 20,000 times a day across 2,000 facilities,” he said.
Development of the algorithm for intracranial hemorrhage is part of an effort by vRAD to make its operations more efficient, using AI to speed diagnosis and, thereby, improve patient care. And that effort is only just getting started.
Eventually the algorithm now in development may evolve to one that points neuroradiologists to specific areas in the CT images where they should look for signs of hemorrhage. And the company has plans to develop algorithms focused on life-threatening conditions besides intracranial bleeds. High on the list are pulmonary embolism and aortic tears. The goal, Werb said, is to “move technology close to the patient” so as to “tap the doctor on the shoulder a lot more rapidly.”
One day the company may completely bridge the gap between exam and interpretation. Working in concert with equipment manufacturers, vRAD hopes to embed AI algorithms in the scanners themselves.
This would allow the algorithms to flag suspicious images — while the patient is still on the scanner table.
Watch the VIDEO “Examples of Artificial Intelligence in Medical Imaging Diagnostics.” This shows an example of how AI can assess mammography images.
Watch the VIDEO “Development of Artificial Intelligence to Aid Radiology,” an interview with Mark Michalski, M.D., director of the Center for Clinical Data Science at Massachusetts General Hospital, explaining the basis of artificial intelligence in radiology.
Editor’s note: This is the first blog in a series of four by industry consultant Greg Freiherr on Working With Smart Machines. To read all of Greg's blogs, click here.