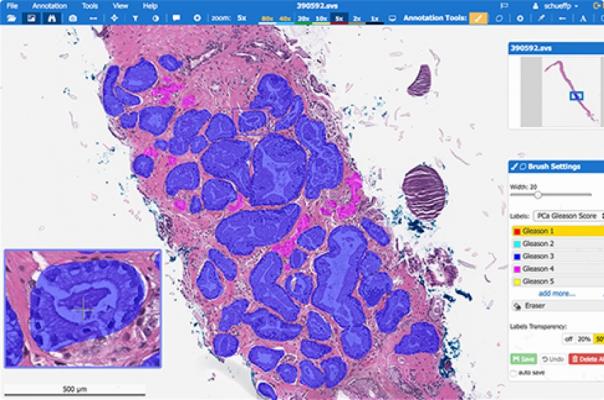
July 16, 2019 — Computational pathology company Paige announced the publication of an article in Nature Medicine describing an artificial intelligence (AI) system for computational pathology that achieves clinical-grade accuracy levels. The paper provides further scientific evidence that pathologists’ work in diagnosing and treating cancer can be complemented and aided through the deployment of computational decision-support systems to improve patient care.
The team of scientists responsible for the work described in the article developed specially-designed deep learning algorithms to build a system that can detect prostate cancer, skin cancer and breast cancer with near-perfect accuracy. These algorithms are based on a vast dataset of nearly 45,000 de-identified, digitized slide images from more than 15,000 cancer patients from 44 countries.
The paper outlines how a series of novel algorithms, created using datasets 10 times larger than those that have been manually curated, performed better and also are more generalizable. The significance of this new development hinges on the fact that curating datasets can be prohibitively expensive and time intensive. By eliminating the need to curate datasets, Paige can now develop many more highly accurate algorithms that can be built into clinical decision support products to help pathologists around the world drive better patient care.
Paige plans to commercialize several of these solutions to address the most pressing needs in pathology to improve patient care. The company has already built on the academic work described in Nature Medicine to develop a clinical product, based on technology currently under review by the U.S. Food and Drug Administration (FDA) as a designated Breakthrough Device, for an intended indication different than the one described in the article.
All data collection, research and analysis for this research was conducted exclusively at Memorial Sloan Kettering (MSK) in New York City. The publication of the study’s findings was the result of collaboration between numerous researchers and clinicians, and made possible by Paige’s partnership with MSK. All data were de-identified and did not contain any protected health information or label text.
Watch the VIDEO: Integrating Digital Pathology With Radiology
For more information: www.nature.com/nm
Reference
1. Campanella G., Hanna M.G., Geneslaw L., et al. Clinical-grade Computational Pathology using Weakly Supervised Deep Learning on Whole Slide Images. Nature Medicine, July 15, 2019. https://doi.org/10.1038/s41591-019-0508-1