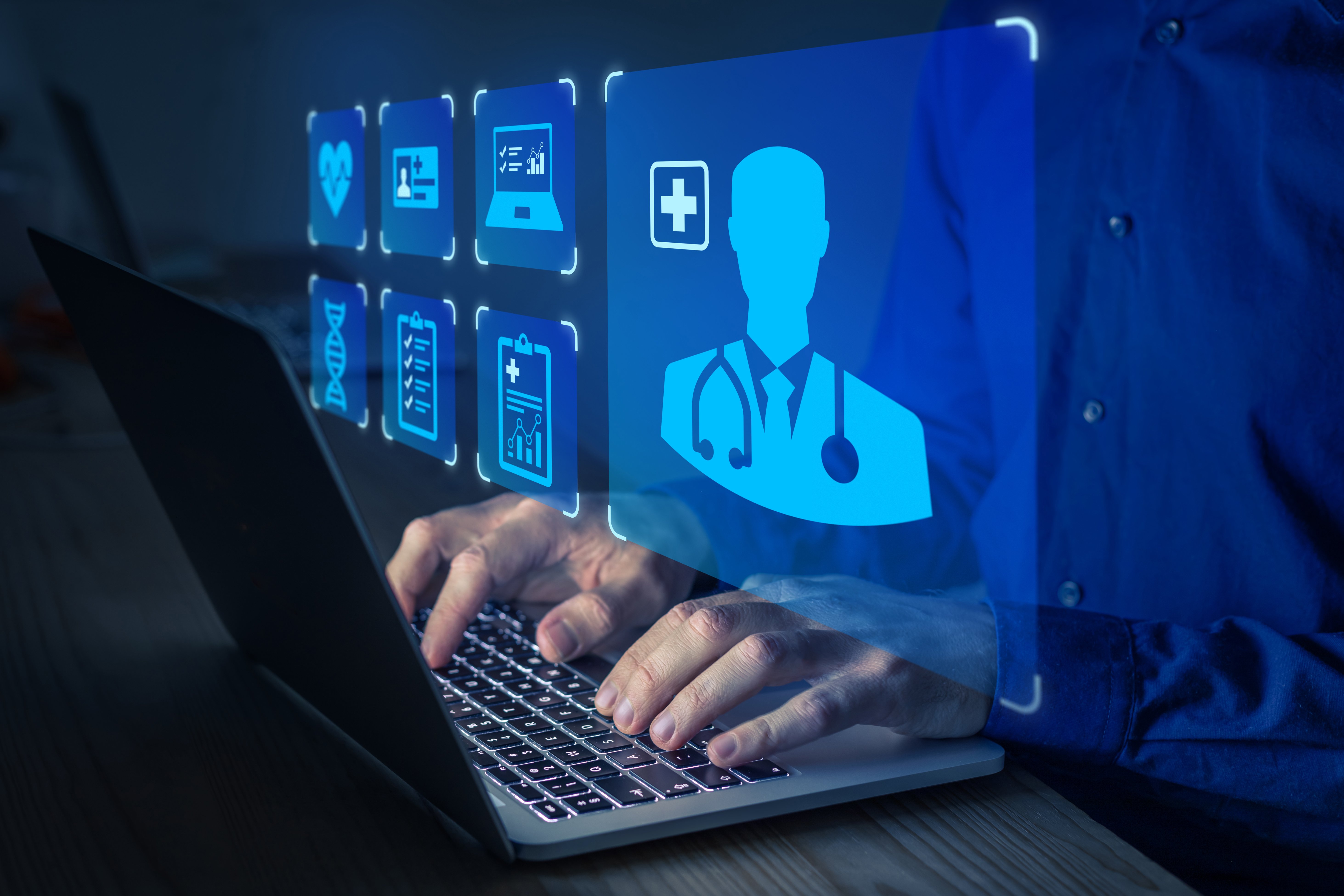
The rapid growth of healthcare data has reached unprecedented heights, making up about 30% of the world’s stored data.¹ Healthcare alone is estimated to generate up to 137 terabytes of data per day,² with both clinical and machine-generated data contributing to this tremendous volume. What’s more, the introduction and adoption of new and valuable healthcare technologies means that healthcare data is continuing to grow by 47% every year.³
Healthcare providers tasked with the monumental task of reviewing, managing and maintaining this data face an untenable challenge. Recent findings from the National Institutes of Health (NIH) indicate a distressing trend in which 12.9% of patient safety incidents are linked to provider stress, with an alarming 30.6% of these incidents resulting in severe harm, prompting urgent investigation.⁴
A substantial portion of provider stress stems not only from this escalating data load, but also from the complex and often cumbersome workflows associated with the capture, discovery and review of clinical information during patient encounters. Moreover, the persistent burden of repetitive data-related tasks is regularly cited as a major contributor to burnout across the entire continuum of care.
Finding a New Approach to Clinical Workflow Design
Addressing these challenges requires a new approach to clinical workflow design. Not only do traditional healthcare workflows suffer from interoperability dilemmas, the inflexible nature of disparate systems and configurations across various vendors also results in disjointed, cumbersome workflows that impede efficiency and require repetitive monotonous administrative work.
Enter micro-workflows — a paradigm shift that dissects intricate tasks into smaller, autonomous steps. Think of assembling a large puzzle: instead of grappling with the entire puzzle at once, micro-workflows strategically divide the task into smaller, more digestible pieces, and all the pieces are already right-side up.
Consider a routine healthcare encounter where a provider captures an image from a mobile device and includes it in a patient’s electronic health record (EHR) as a macro-workflow. In contrast, micro-workflows deconstruct this process into discrete steps, for example, identifying the patient within the EHR, capturing the image, inputting relevant metadata from the encounter, and seamlessly sharing the image with the patient’s care team, when necessary.
These seemingly small efforts may not sound burdensome for a single patient interaction, but when multiplied by dozens of similar interactions each day, these repetitive tasks decrease the amount of time that providers have for meaningful patient interaction, lower overall productivity, increase the likelihood of human error, and contribute significantly to burnout and fatigue.
Benefits of a Micro-workflow Approach
Adopting a micro-workflow approach (think deconstructed workflow), enables targeted attention, facilitates automation, and offers flexibility for individual component modification or enhancement without causing disruptions to the entire process. This effectively enables a strategy for rendering complex healthcare workflows adaptable, efficient, and responsive to evolving clinical requirements and technological advancements.
This revolutionary approach to discrete task segmentation and automation expedites processes, mitigates burnout, and fosters precision in diagnosis and reporting. Moreover, these workflows bridge disparate data sources, culminating in a unified view in which to identify trends and anomalies to provide better clinical insights and optimize key cost performance indicators.
Effectively breaking down complex traditional clinical processes into micro-workflows requires an imaging ecosystem orchestrator that can isolate and automate tasks across clinical, IT and artificial intelligence (AI) applications. Examples of micro-workflows in action include orchestration and monitoring of AI algorithms that assess and prioritize imaging studies according to clinical urgency (e.g. stroke cases) to significantly reduce response time. They also enable real-time EHR queries to support encounters-based imaging workflow, like point-of-care ultrasound, to automatically associate imaging data with a patient chart and support billing workflows. There are also opportunities to automatically monitor and model all user interactions across multiple vendor applications to visualize relationships between the workflow, applications, and associated infrastructure and enable proactive remediation of potential performance issues.
Identifying and Automating Micro-workflows
The ability to identify and automate micro-workflows represents the next step in the evolution of healthcare digital transformation. Healthcare organizations struggling with complex imaging systems, growing data volumes, and disjointed clinical workflows have been seeking imaging ecosystem orchestration tools that bridge the gap between disparate data sources to more effectively analyze and model billions of data points. In doing so, they are better positioned to facilitate meaningful optimization throughout the enterprise and enable real-time access, interoperability, and comprehensive monitoring the entire healthcare IT application portfolio — regardless of vendor — through a single pane of glass.
Jef Williams is president of Paragon Health IT, a Sacramento, Calif., based healthcare IT consulting group.
REFERENCES:
1. OECD (2019), Health in the 21st Century: Putting Data to Work for Stronger Health Systems, OECD Health Policy Studies, OECD Publishing, Paris, https://doi.org/10.1787/e3b23f8e-en.
2. HealthTech Magazine. “Structured vs. Unstructured Data in Healthcare.” HealthTech Magazine, May 2023, https://healthtechmagazine.net/article/2023/05/structured-vs-unstructured-data-in-healthcare-perfcon#
3. Ibid.
4. Marra AR, Algwizani A, Alzunitan M, Brennan TMH, Edmond MB. Descriptive Epidemiology of Safety Events at an Academic Medical Center. Int J Environ Res Public Health. 2020 Jan 4;17(1):353. doi: 10.3390/ijerph17010353. PMID: 31947963; PMCID: PMC6982027.