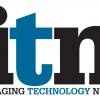
Greg Freiherr has reported on developments in radiology since 1983. He runs the consulting service, The Freiherr Group.
AI and Innovation: When Intelligence is No Longer “Artificial”
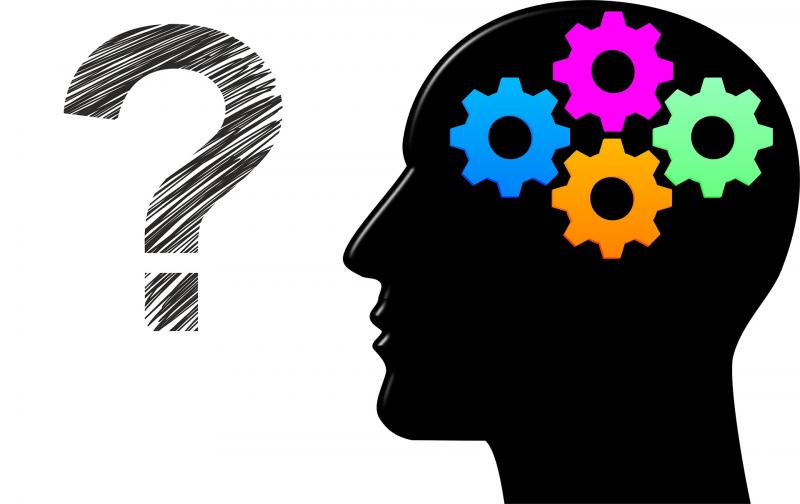
Like the industrial revolution, which led to wrenching changes in society (for example, factory robotics and automatic pinsetters at the ends of bowling alleys), the widening use of artificial intelligence (AI) will change the American workforce. We are only seeing the ripples of what may turn into the wake of major innovation.
The last time something like this happened in radiology was 40 years ago with positron emission tomography (PET) and magnetic resonance imaging (MRI). Since then we have adopted small innovations and pretended they were big. Artificial intelligence could force radiology to break with that.
But what will we call this? AI might not be the right term. Machine learning is better. And it’s more than semantics.
What’s In A Word
The meaning of words change over time. Remember when “dialing” meant calling on the phone? Remember when the phone wasn’t called a “landline?” Remember when the phone was for talking?
A GPS app on my smartphone tells me how to get to from one place to another. Double-clicking its side button brings up a high-res camera. I type notes into a word processor; record reminders to myself on a digital recorder app. I’ve stopped wearing a watch. (The time display on my phone covers a third of the display.)
The next logical step is a phone that learns.
Wise people credit their success to surrounding themselves with smart people. Someday I’d like to say the same about my machines.
Classifying “AI” as machine learning will help buoy the argument that intelligent machines are assistants, not replacements.
Flies In The Soup
But there’s a problem. It has to do with manufacturers making money. Machines that learn may not become obsolete very easily. And planned obsolescence is important. Take the light bulb, for example.
Demonstrating the folly of a long-lasting light bulb is the one made more than a century ago by the Shelby Electric Co. of Ohio. It’s been turned off only a handful of times. Yet that bulb is now in its 117th year of illumination. The town of Livermore, Calif., celebrated the bulb’s 1 million hours of operation in 2015, according to the Centennial Bulb website.
If all light bulbs were built to last a century or longer, comparatively few would have been sold. And that is the underlying problem with AI (aka machine learning).
How do you update machines that learn? Improved processors? Maybe. Better learning ability? Perhaps. But if I had a machine that constantly got better at doing what I needed it to do, why would I trade it in or even update it?
The makers of learning machines will solve this problem. A less surmountable barrier, however, is difficulty building machines that will actually add value to medicine. To do that, people have to get involved. And there is the real problem. Physicians and patients will have to be convinced that learning machines are worth the risk; that they can be built and used without risking the future of humankind. Some of that persuasion is already in the works.
Siri, Alexa and Cortana are reshaping the ways people interact with computers and, in the process, how we think about computers. Further changing our views of computers are virtual and augmented realities, which deliver information when and where needed. Whether the public will ultimately embrace machine learning as it relates to medical practice, however, is anything but certain.
Look no further than GMOs (genetically manipulated organisms) for an example of how something with enormous potential can flounder. The controversy swirling around GMOs has impeded the acceptance of what decades ago was supposed to bring an unprecedented abundance of food. The core concern of so-called Frankenfoods — their safety — continues to be debated. As stated in a New York Times story in April 2018, some consumers seem “terrified of eating an apple with an added anti-browning gene or a pink pineapple genetically enriched with the antioxidant lycopene.”
It is sobering to note that GMO fears are still theoretical. And yet, they have been stopped in their tracks. The bottom line is that GMO foods can never be proven safe. They can only be shown to present no hazard, as of yet. Ditto for AI.
Making IntelligentMachines Palatable
The adoption of machine learning in medicine will only occur with “baby steps.” A crucial one is making these machines palatable to mainstream radiologists.
To do so, safeguards must be put in place to ensure that learning machines are designed only to help. Doing so will go a long way toward alleviating the fear surrounding AI today.
The second crucial “baby step” involves demonstrating value. Learning machines must deliver on the promise of value-based medicine. They must help improve patient care (possibly measured by patient outcomes), and boost efficiency and cost effectiveness.
The third step that needs taking: Learning machines have to be shown to promote patient engagement in healthcare. Maybe this will happen by helping patients live healthier. Or maybe by providing more time for physicians to spend with their patients, taking on time consuming burdens or helping in communicating difficult concepts. There are lots of possibilities.
The takeaway is that learning machines have to demonstrate value in the humdrum metrics that now characterize the practice of medicine. And they have to be usable.
I can imagine a time when learning machines are distributed across multiple devices — tablets and desktops, smartphones and TVs, maybe even dedicated boxes like Amazon Echoes. Each will use a voice interface to promote efficiency with providers and patients. And, as they learn what we need, we get more efficient. And that has to be provable.
This future will happen only with the coming together of different but complementary technologies, along with a public recognition that these technologies are making a positive difference. Development has to be done cautiously and safely, with benefits proven and documented along the way. Otherwise fear will win out.
And AI will go the way of another acronym now associated more with Frankenstein than progress.