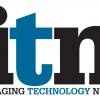
Greg Freiherr has reported on developments in radiology since 1983. He runs the consulting service, The Freiherr Group.
What “Dark Star” Has To Say About AI In Radiology
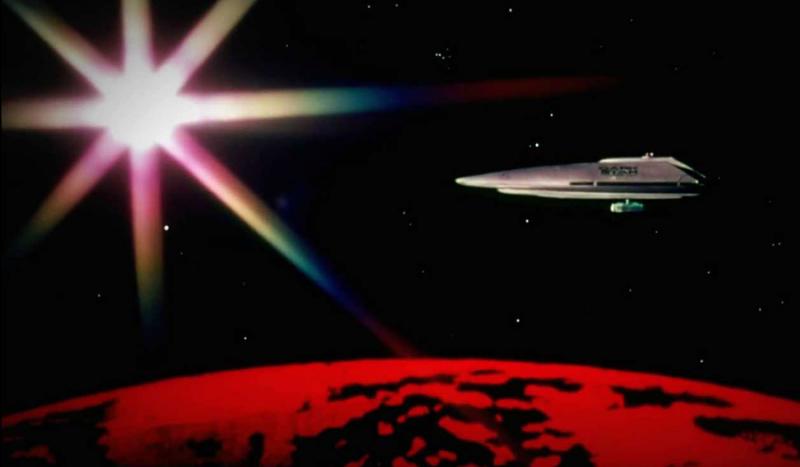
Image courtesy of Fandor
Doolittle: What is your one purpose in life?
Bomb: To explode, of course.
Doolittle: And you can only do it once, right?
Bomb: That is correct.
Doolittle: And you wouldn’t want to explode on the basis of false data, would you?
Bomb: Of course not.
Doolittle: Well then…
— Exchange between Dark Star Crewman Doolittle as he tries to convince an artificially intelligent thermostellar device (Bomb #20) to return to the bombay
In the movie “Dark Star,” a spaceship crewman does his best to talk an artificial intelligence (AI) thermostellar device out of exploding. Bomb #20, as it is named, retorts that it has received the deployment signal and that its countdown to detonation has begun. The exchange between Doolittle and the Bomb illustrates that, just because an analysis is actionable, it does not necessarily mean it should be acted upon. (This is especially so given that the signal came from a faulty communications relay that should have been fixed by the crew.) Machine learning algorithms can find patterns in huge volumes of data, volumes that might overwhelm individuals or teams of human analysts. But their value depends on what is done with them. Here people are the weak link. And the countering force is inertia in human behavior. People must act on the insights; change the way they have been doing things; change workflow. But how should that workflow be changed? The real challenge, consequently, may not be as much getting the insight as it is applying it. And that is where automation has come in. Engineers have been automating imaging equipment for years. The idea has always been to increase reproducibility by decreasing dependence on the skills of the operator. Automation has been particularly evident in ultrasound, where image optimization algorithms have been in vogue for years. Artificial intelligence raises new questions about how far automation can — and should — go. Delegating the implementation to AI may not be the way to go, because it assumes that artificially intelligent machines “think” the way people do. In “Dark Star,” Bomb #20 misunderstands what Doolittle says, rapidly develops a god complex, and dispels the darkness of space with an explosion of light, killing the onboard crew. Admittedly, science fiction tends to focus on the darker side of AI. But its cautionary tales should not be dismissed outright.
“Dark Star” History Made in 1974, “Dark Star” has been all but forgotten in the film world. Yet the dramatic moments in this cult favorite are relevant to what is happening as the imaging community grapples with the increasing load of clinical data and the prospect of AI as a remedy. For those dealing with information technology, a lyric — “the years move faster than the days” — in the “Dark Star” theme song, entitled “Benson Arizona,” stands out. Despite the seeming urgency of data captured daily, progress toward their use drags on. At the same time, deadlines — once months or years in the future — accelerate stealthily until suddenly they are there. AI has the potential to change that. Smart algorithms today are autopositioning patients, reducing the effort — and skill — needed from technologists. In concert with cloud computing, they are simplifying complex exams, such as cardiac magnetic resonance imaging (MRI), slashing what were one-hour exam times by two-thirds or more. And new smart algorithms are on the way. At RSNA 2017, multimodality vendors will feature a wide variety of smart algorithms. Some have been developed in-house; some by suppliers, such as Arterys. This company provides GE Healthcare with the AI muscle to run its ViosWorks, a cloud and AI driven application that GE says provides — through cardiac MR — “detailed quantitative flow, regurgitant measurements and stroke volume.” This year the company, from the RSNA exhibit floor, will describe how its AI-based medical imaging platform can “help doctors diagnose heart problems in 15 seconds.” Other companies will make similarly startling claims. One of the most startling will come from an Israeli startup AIDOC (artificial intelligence doctor) Medical, which plans to show how its AI can enhance workflow by giving the radiologist a preview of “what is most relevant for interpretation.”
Theme Song As Location This fall I swept through the American Southwest on a month-long camping trip that had Benson, Ariz., at its southern most tip. There, in this town of 5,000, on Fifth Street, next to Mary Ann’s Mostly Books, across from a vacant lot, was Elliot’s. Its barbers, reviewed on Facebook as the “friendliest in Cochise County,” lived up to the claim. On one wall was a picture of Jack Nicholson getting a haircut (although not at Elliot’s); on a corner shelf a model of Batman held two flags (one North Korean; the other modeled after flags carried in gay pride parades). Elliot’s barbers told the stories behind each, expanding our conversation into politics and politicians, as one of them snipped away at my shock of hair. They spoke of fundamental beliefs based on how they viewed the world’s problems and their solutions. I was struck by the relevance of what they said. (As Dark Star crewmate Doolittle said to Bomb #20, “the concept is valid no matter where it originates.”) Unquestionably the imaging world will become increasingly complex. It is already generating humanly incomprehensible volumes of data. In the months and years ahead, pressure will mount to manage these data with smart tools just as people will yearn for simpler days. Inevitably, they will seek to apply sophisticated technologies to accomplish simplistic goals. Nothing could be more dangerous. As AI evolves, so must human intelligence. We can’t rely on “smart shovels” to dig gold nuggets from mountains of data without understanding how those nuggets should be used. And our thinking about how to use smart tools must evolve with the technology. The disconnects between artificial and human intelligence are already taking shape. Insights gained from AI dives into big data are being turned into “alerts,” too many of which can cause “alert fatigue,” leading operators to override them. Someone has to decide what patterns or insights are so important that they need to be acted upon — and how to act upon them. This process must begin by answering why the algorithm was developed in the first place. From the very start in the R&D process, the developer must explain why the analysis is needed and understand how it should be used. That will require developers and providers to get closer than they have ever been before. Human collaboration may be the most significant challenge of AI, one that must be met to make sure that what machines do is being done for the benefit of the patient and provider.
|