WEBINAR: Multi-Modality 3-D Quantitative Imaging in Cancer Care: Clinical Value and Future Perspectives
This webinar is supported by an educational grant from Philips Healthcare
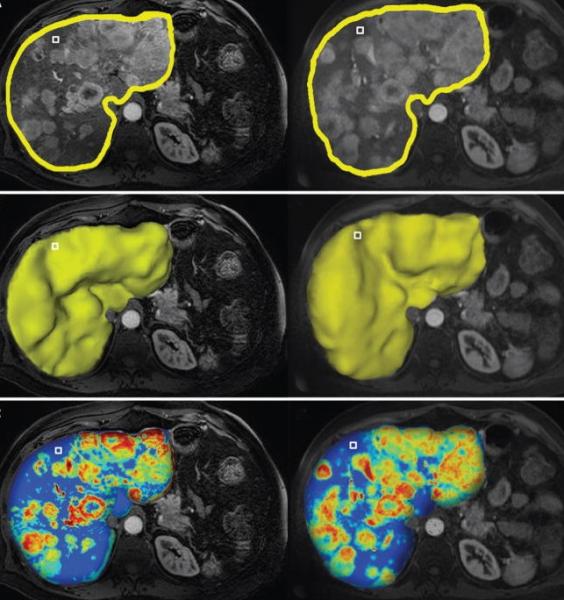
The webinar "Multi-Modality 3D Quantitative Imaging in Cancer Care: Clinical Value and Future Perspectives" focuses on the role of image analysis and artificial intelligence for image-guided, minimally invasive cancer therapies. It introduces the audience to the mechanisms and principles of image analysis, outlines the growing role of machine learning for the therapeutic algorithm and the decision making processes in interventional oncology.
The webinar took place Oct. 3, 2017. Watch the recorded archive version of this webinar by registering.
Register to watch the archive version of this webinar
Statement of Purpose
Liver cancer is the second most common cause of cancer-related death worldwide and most cases are diagnosed at intermediate to advanced stages of the disease, making most patients no longer amenable to surgical therapies. Minimally invasive, loco-regional image guided therapies, such as chemoembolization, have become the mainstay therapy for such patients. These image-guided interventions also gave birth to the new field of interventional oncology, a subspecialty of interventional radiology which is increasingly considered as the new and fourth pillar of cancer care (next to medical, surgical and radiation oncology). The explosive growth of such therapies requires new and more efficient intra- and post-procedural imaging solutions. This webinar will focus on the role of image analysis and artificial intelligence for image-guided, minimally invasive cancer therapies and introduce the audience to the mechanisms of action, principles of image analysis and the growing role of machine learning for the therapeutic algorithm and decision making in interventional oncology.
Learning Objectives
Upon completion of this activity, particpants will be able to:
• Summarize the principles and applications of image-guided minimally invasive tumor therapy.
• Describe the role of cancer imaging and multi-modality tumor tracking for local therapies of liver cancer.
• Review novel software-assisted 3-D quantitative tools to evaluate surrogate endpoints of therapeutic efficacy.
• Explain the growing role of machine learning in the automation and standardization of image analysis.
Intended Audience:
This activity is intended for oncologists, radiologist, nurse practitioners, biomedical engineers, and other clinicians interested in the management of liver cancer.
Presenter
Julius Chapiro, M.D., Ph.D.
Research Scientist and Resident Physician
Yale School of Medicine
Dr. Chapiro is a research scientist at the Department of Radiology and Biomedical Imaging, Yale University School of Medicine. After graduating from the University of Leipzig and upon completion of his research thesis at the Justus-Liebig University in Giessen, he served as a postdoctoral fellow in interventional oncology at The Johns Hopkins Hospital and then as radiology resident at the Department of Radiology, Charité University Hospital in Berlin.
Dr. Chapiro works on the development of novel imaging biomarkers, tumor response criteria, staging systems and molecular imaging techniques for image-guided liver cancer therapies. His translational research portfolio includes the development of novel embolic agents as well as the application of artificial intelligence and machine learning solutions for the assessment of liver cancer. His basic research interest mainly focuses on tumor metabolism and immuno-oncology. With over 50 peer-reviewed publications, book chapters and news articles, Dr. Chapiro advocates the element of personalized medicine in interventional oncology, which is the most rapidly growing field in interventional radiology practice. Creating innovative and clinically practicable solutions and translating them from concept to practice has been his central mission for the past five years. He is the co-director of the Yale Radiology Research laboratory.