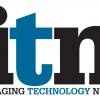
Greg Freiherr has reported on developments in radiology since 1983. He runs the consulting service, The Freiherr Group.
Smart Scanners: Will AI Take the Controls?
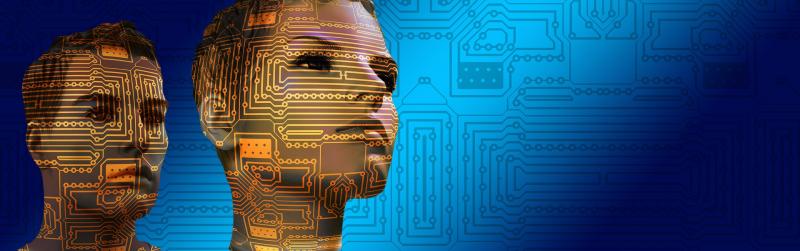
Image courtesy of Pixabay
Go back to the early 1990s and you’ll come across a brilliant, yet fatally flawed, idea. It was called “Evolving Images,” introduced by the Israeli company Elscint as a feature of its high-end magnetic resonance (MR) scanners.
The idea was brilliant in that the software constructed images as data became available. Doing so reduced the risk of motion artifact, which was a big problem in the early days of MR due to long scan times. The rationale was that the scan could be stopped, when the image reached diagnostic quality. This reduced patient discomfort and, consequently, the chance that the patient would move and ruin the image.
The idea was flawed because Elscint failed to recognize that radiologists were not in the control room to see the evolving images. Technologists were. And technologists were not going to stop an exam early and risk being wrong.
Fast forward to the present, where an interesting twist is being put on this idea, one that promises to increase the diagnostic value of medical images and boost productivity.
What if intelligent algorithms were to stand-in for radiologists? And, what if these smart algorithms could guide the scanner to enhance the visualization of a pathology, while ensuring that diagnostic images were produced in the least amount of time?
Would radiologists sign off on the idea? Would administrators? Would the patient? Would the FDA?
This modern adaptation of the Evolving Images concept is being kicked around now at Merge, which IBM acquired earlier this year. Most of the attention given this corporate acquisition has centered on how artificially intelligent algorithms, built into Big Blue’s Watson Health, might learn to help physicians come up with diagnoses (see Radiology Faces Frightening New World).
But Merge’s chief strategy officer, Steven Tolle, told me at RSNA that this is just one possibility. Deep learning algorithms might be incorporated into scanners, MR scanners, for example. Once onboard they could tap into the stream of incoming image data, looking for signs of disease and — when such signs are found — adjust the machine to optimize the scan.
“Think about a smart modality,” Tolle said. “You have a patient in the MR machine and you do a scout. And you run that scout image through Watson and Watson says, ‘Oh, wait, let’s look over here and take this view of the patient.’ ”
It’s not so far-fetched. Right now many ultrasound systems automatically adjust image parameters on the fly to produce images according to the preferences of their operators or the physicians who interpret them. In fact, there is not a single modality that doesn’t use automation to reduce exam time and boost productivity in some way. The gamut runs from Automatic Exposure Control software in radiography to algorithms that set certain computed tomography (CT) parameters on the basis of scout scans.
Just as Elscint engineers created scanners 20 years ago that displayed “evolving” MR images, so might deep learning algorithms be embedded in future MR scanners to adjust the parameters of the scan based on accumulating information.
And that’s just MR. What if AI could be embedded in CT scanners to look for signs of disease outside the area on which radiologists are focused? A head-to-toe CT scan might be performed, for example, following a car crash. The greatest concern may be injury due to blunt force trauma. But a smart CT scanner could flag evidence of pulmonary nodules or lung cancer.
And it need not be done at the level of the scanner. AI analyses might be run routinely in the background when the images are sent for archive.
Regardless of where or how deep learning algorithms are applied, there is definitely a need. Diseases might be found early and treatment started when it has the best chance to be effective.
Further, DL might mitigate the legal liability that arises when a patient, scanned for one reason and given a clean bill of health, later develops an entirely different disease — and the scans done for another reason are examined and found to have signs of that disease.
If only the doctors had known where to look goes the old refrain. Soon they may.
But there are issues. Lots of issues. Foremost is that asymptomatic abnormalities may not develop into disease. Spotting — and acting upon — any and all signs of disease can easily lead to over treatment, and adverse side effects on patients, as well as spiraling healthcare costs.
There are also concerns about whether machines should be allowed to interpret medical data. The concerns are less, when they are used as disease spotters, directing physicians’ attention to the possible locations of disease in medical images that have been archived and otherwise would not be examined. But should they be allowed to adjust or guide the performance of scanners in real time? And if so, to what degree and with what input from the physician?
Tolle said the issue must be addressed in the design of the machine. Smart machines, he said, must always be subservient to the physician.
“So long as we focus on the machine being an assistant that helps the physician, we are OK,” he said. “The minute we start talking about physicians not being the driver, then there is a problem.
And that is not where we are headed.”
Editor’s note: This is the second blog in a series of four by industry consultant Greg Freiherr on Machine Learning and IT. The first blog, Will the FDA Be Too Much for Intelligent Machines?, can be found here.