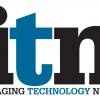
Greg Freiherr has reported on developments in radiology since 1983. He runs the consulting service, The Freiherr Group.
Beyond Diagnostics: Data Mining With AI
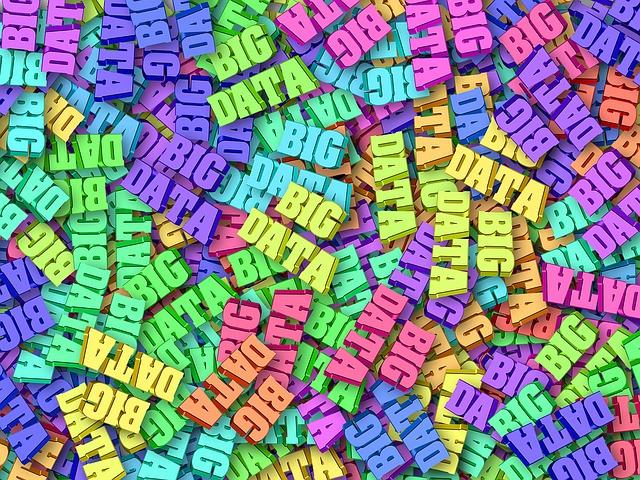
Image courtesy of Pixabay.
Statistics have been the basis of modern medical practice. Soon it will be Big Data’s turn.
In today’s digital world, where just about everything medical from heartbeats to steps to blood sugar is measured, the relevance of statistics has been called into question. With millions, billions, even trillions of data points, people cannot possibly plumb the true depths of medical well-being.
The challenge is not just choosing which data points to examine but how to combine different types of data points to draw truly relevant conclusions. How can we choose best practices when it is impossible to be sure which metrics are needed to identify those practices?
Not long ago, it was hoped that computed tomography (CT) and magnetic resonance imaging (MRI) might be used to gauge patient response to radiation or chemotherapy. Physicians opined that the documented shrinkage of tumors might be an indicator of therapeutic efficacy. Molecular imaging has shown, however, that tumor activity, defined by standard uptake values, may be a better indicator of therapeutic response. Yet experience with the use of SUVs has identified certain variables that may throw off their accuracy — for example, the protocols used to measure tumor activity and tracer dosage; even more fundamentally, the scanner itself and the sophistication of its technology. A better way to determine patient response may be a comprehensive examination of patient data, one that involves hundreds or thousands of data points.
Such analyses will require technologies that are only beginning to emerge. Deep learning (DL) algorithms, an advanced form of artificial intelligence (AI), promise to identify specific practices — the use, for example, of a certain modality to make specific measurements. These might improve patient outcomes by triggering the early application of certain types of treatment.
Data mining could provide the basis for not just the accurate assessment of patients but the prediction of what they need to become and even stay healthy. In short, AI might finally deliver on the promise of personalized medical care.
But many issues are still unresolved. For example, when assessing medical images, will these algorithms recognize artifacts for what they are — the debris of less-than-perfect technologies or techniques? For that matter, how will they handle images of varying quality?
One of the strengths often cited by proponents of DL algorithms is their self-teaching nature. Proponents argue that this will allow them to draw conclusions free from past biases, providing them the ability to uncover patterns that previously had been invisible. But this very premise may be flawed. Medical schools, for example, don’t unleash their freshmen on cadavers and have them “learn.” While starting from scratch may lead to novel observations, some of those observations could be wrong.
The analysis of Big Data by DL algorithms, therefore, could in some situations actually reduce the effectiveness of medical care and increase costs, a risk that is especially likely if erroneous Big Data analyses lead to the widespread adoption of best practices.
While the promise of Big Data mining is great, development of AI-based mining tools must be done carefully, as must their application. Similarly physicians must be careful not to believe too much in the ability of such data mining. Whatever patterns DL algorithms may uncover, their value must be judged in the context of the medical education and experience that made human physicians what they are.
Editor’s note: This is the fourth blog in a series of four by industry consultant Greg Freiherr on Working With Smart Machines. You can read the first blog, Will Artificial Intelligence Find a Home in PACS?, here; his second blog, “Watson, Come Here … Radiology Needs You,” here; and his third blog, “Artificial Intelligence: Promise or Pitfall for Radiology?” here.